Dobb·E
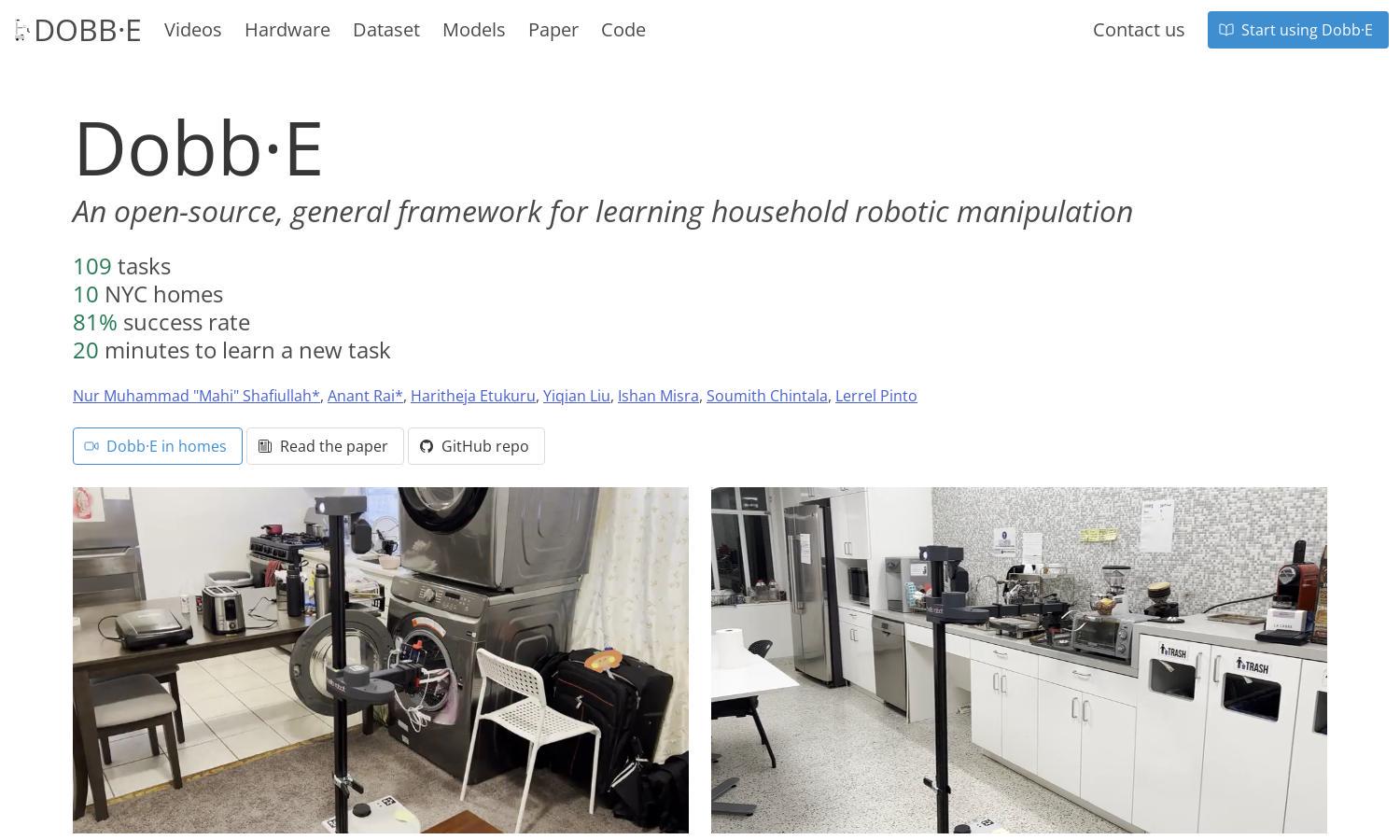
About Dobb·E
Dobb·E is a pioneering open-source framework aimed at enhancing household robotics. Targeted at researchers and developers, it enables robots to learn tasks via imitation learning in just 20 minutes. Its unique feature, "The Stick," allows for quick and affordable data collection, solving home automation challenges.
Dobb·E is open-source and free to use, promoting accessibility in robotics research. Users can access various tools and datasets without a subscription. Premium features and enhancements are still in development but planned for future releases, ensuring continual improvement and user engagement.
Dobb·E boasts a user-friendly interface, designed to facilitate easy navigation through tools, datasets, and documentation. The streamlined layout and intuitive features enhance the user experience, making it simple for developers and researchers to engage with tasks and resources essential for home robotics innovation.
How Dobb·E works
Users start by onboarding Dobb·E, where they can access the necessary tools, like "The Stick," to collect task demonstrations in just five minutes. After gathering data, they can train their robot using the Home Pretrained Representations (HPR). Users adapt the model within minutes to tackle new tasks efficiently, enhancing their robotics projects easily.
Key Features for Dobb·E
Imitation Learning with Ease
Dobb·E's unique imitation learning capability allows robots to learn household tasks quickly and effectively. This feature exemplifies how Dobb·E empowers users with versatile robotic training methods, significantly reducing the time needed to teach robots new skills, enhancing productivity in home automation efforts.
Affordable Data Collection Tool
The Stick is Dobb·E's innovative demonstration collection tool, built using inexpensive materials. This key feature democratizes access to data collection for robotic training, enabling users to gather valuable task demonstrations seamlessly, ultimately facilitating smoother learning processes and reducing barriers in home robotics research.
Home Pretrained Representations (HPR)
Dobb·E’s Home Pretrained Representations (HPR) are pivotal, allowing users to initialize robot policies for new tasks effectively. HPR enhances the model's adaptability and learning efficiency in diverse home environments, informing Dobb·E's success rates and its overall value in household robotics applications.
You may also like:
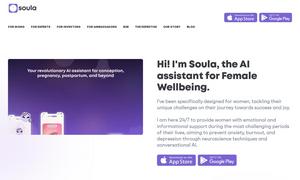